Comparative Evaluation of Sentiment Analysis Methods Across Arabic Dialects
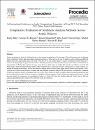
View/ Open
Publisher version (Check access options)
Check access options
Date
2017Author
Baly, RamyEl-Khoury, Georges
Moukalled, Rawan
Aoun, Rita
Hajj, Hazem
Shaban, Khaled Bashir
El-Hajj, Wassim
...show more authors ...show less authors
Metadata
Show full item recordAbstract
Sentiment analysis in Arabic is challenging due to the complex morphology of the language. The task becomes more challenging when considering Twitter data that contain significant amounts of noise such as the use of Arabizi, code-switching and different dialects that varies significantly across the Arab world, the use of non-Textual objects to express sentiments, and the frequent occurrence of misspellings and grammatical mistakes. Modeling sentiment in Twitter should become easier when we understand the characteristics of Twitter data and how its usage varies from one Arab region to another. We describe our effort to create the first Multi-Dialect Arabic Sentiment Twitter Dataset (MD-ArSenTD) that is composed of tweets collected from 12 Arab countries, annotated for sentiment and dialect. We use this dataset to analyze tweets collected from Egypt and the United Arab Emirates (UAE), with the aim of discovering distinctive features that may facilitate sentiment analysis. We also perform a comparative evaluation of different sentiment models on Egyptian and UAE tweets. These models are based on feature engineering and deep learning, and have already achieved state-of-The-Art accuracies in English sentiment analysis. Results indicate the superior performance of deep learning models, the importance of morphological features in Arabic NLP, and that handling dialectal Arabic leads to different outcomes depending on the country from which the tweets are collected.
Collections
- Computer Science & Engineering [2426 items ]