An innovative deep anomaly detection of building energy consumption using energy time-series images
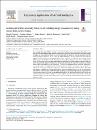
View/ Open
Publisher version (Check access options)
Check access options
Date
2023-01-06Author
Abigail, CopiacoHimeur, Yassine
Amira, Abbes
Mansoor, Wathiq
Fadli, Fodil
Atalla, Shadi
Sohail, Shahab Saquib
...show more authors ...show less authors
Metadata
Show full item recordAbstract
Deep anomaly detection (DAD) is essential in optimizing building energy management. Nonetheless, most existing works concerning this field consider unsupervised learning and involve the analysis of sensor readings through a one-dimensional (1D) energy time series, which limits the options for detecting anomalies within the building’s energy consumption. To the best of the authors’ knowledge, this paper presents the first study that explores using two-dimensional (2D) image representations as features of a supervised deep transfer learning (DTL) approach. Specifically, using 2D image representations allows taking advantage of any underlying data within the feature set, providing more possibilities to encode data and detect pertinent features which may not be considered in standard 1D time-series. Furthermore, the effects of using CNN activations as machine learning (ML) model features are also investigated to combine the advantages of both techniques. Additionally, the concept of layer and hyperparameter variation for the CNN model is also studied, with the objective of reducing the overall time computation and resource requirements of the proposed system. Hence, this makes our approach a candidate for edge-based applications. As per the conducted experiments, the top methodology rests at the F1-scores of 93.63% and 99.89% under simulated and real-world energy datasets, respectively. This involves using grayscale 2D image representations that combine CNN activations extracted from AlexNet and GoogleNet pre-trained models as features to a linear support vector machine (SVM) classifier. Finally, the comparison analysis with the state-of-the-art has shown the superiority of the proposed method in various assessment criteria.
Collections
- Architecture & Urban Planning [307 items ]