Convolutional Sparse Support Estimator-Based COVID-19 Recognition from X-Ray Images
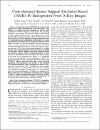
Date
2021Author
Yamac M.Ahishali M.
Degerli A.
Kiranyaz, Mustafa Serkan
Chowdhury M.E.H.
Gabbouj M.
...show more authors ...show less authors
Metadata
Show full item recordAbstract
Coronavirus disease (COVID-19) has been the main agenda of the whole world ever since it came into sight. X-ray imaging is a common and easily accessible tool that has great potential for COVID-19 diagnosis and prognosis. Deep learning techniques can generally provide state-of-the-art performance in many classification tasks when trained properly over large data sets. However, data scarcity can be a crucial obstacle when using them for COVID-19 detection. Alternative approaches such as representation-based classification [collaborative or sparse representation (SR)] might provide satisfactory performance with limited size data sets, but they generally fall short in performance or speed compared to the neural network (NN)-based methods. To address this deficiency, convolution support estimation network (CSEN) has recently been proposed as a bridge between representation-based and NN approaches by providing a noniterative real-time mapping from query sample to ideally SR coefficient support, which is critical information for class decision in representation-based techniques. The main premises of this study can be summarized as follows: 1) A benchmark X-ray data set, namely QaTa-Cov19, containing over 6200 X-ray images is created. The data set covering 462 X-ray images from COVID-19 patients along with three other classes; bacterial pneumonia, viral pneumonia, and normal. 2) The proposed CSEN-based classification scheme equipped with feature extraction from state-of-the-art deep NN solution for X-ray images, CheXNet, achieves over 98% sensitivity and over 95% specificity for COVID-19 recognition directly from raw X-ray images when the average performance of 5-fold cross validation over QaTa-Cov19 data set is calculated. 3) Having such an elegant COVID-19 assistive diagnosis performance, this study further provides evidence that COVID-19 induces a unique pattern in X-rays that can be discriminated with high accuracy.
Collections
- COVID-19 Research [834 items ]
- Electrical Engineering [2647 items ]
Related items
Showing items related by title, author, creator and subject.
-
Encoder-decoder architecture for ultrasound IMC segmentation and cIMT measurement
Al-Mohannadi A.; Al-Maadeed, Somaya; Elharrouss O.; Sadasivuni K.K. ( MDPI , 2021 , Article)Cardiovascular diseases (CVDs) have shown a huge impact on the number of deaths in the world. Thus, common carotid artery (CCA) segmentation and intima-media thickness (IMT) measurements have been significantly implemented ... -
ANFIS-Net for automatic detection of COVID-19
Al-ali A.; Elharrouss O.; Qidwai U.; Al-Maadeed, Somaya ( Nature Research , 2021 , Article)Among the most leading causes of mortality across the globe are infectious diseases which have cost tremendous lives with the latest being coronavirus (COVID-19) that has become the most recent challenging issue. The extreme ... -
Computer aided diagnosis system based on machine learning techniques for lung cancer
Al-Absi, Hamada R. H.; Samir, Brahim Belhaouari; Shaban, Khaled Bashir; Sulaiman, Suziah (2012 , Conference Paper)Cancer is a leading cause of death worldwide. Lung cancer is a type of cancer that is considered as one of the most leading causes of death globally. In Malaysia, it is the 3rd common cancer type and the 2nd type of cancer ...