Machine learning-based shear capacity prediction and reliability analysis of shear-critical RC beams strengthened with inorganic composites
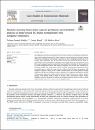
View/ Open
Publisher version (Check access options)
Check access options
Date
2022Metadata
Show full item recordAbstract
The application of inorganic composites has proven to be an effective strengthening technique for shear-critical reinforced concrete (RC) beams. However, accurate prediction of the shear capacity of RC beams strengthened with inorganic composites has been a challenging problem due to its complex failure mechanism and the interaction between the internal and external shear reinforcements. Besides, the predictive capabilities of the existing models are not satisfactory. Thus, this research proposed machine learning (ML) based models for predicting the shear capacity of RC beams strengthened in shear with inorganic composites, for the first time, considering all important variables. The results of the analyses evidenced that the proposed ML models can be successfully used to predict the shear capacity of shear-critical RC beams strengthened with inorganic composites. Among the ML models examined herein, the extreme gradient boosting (xgBoost) model showed the highest prediction capability. The comparison among the predictions of the proposed xgBoost and existing models evidenced that the efficacy of the xgBoost model is superior to the existing models in terms of accuracy, safety, and economic aspects. Finally, reliability analysis is performed to calibrate the resistance reduction factors in order to attain target reliability indices of 3.5 and 4.0 for the proposed model. 2022 The Authors
Collections
- Civil and Environmental Engineering [869 items ]