An enhanced ensemble deep random vector functional link network for driver fatigue recognition
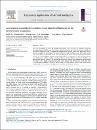
View/ Open
Publisher version (Check access options)
Check access options
Date
2023Author
Li, RuilinGao, Ruobin
Yuan, Liqiang
Suganthan, P.N.
Wang, Lipo
Sourina, Olga
...show more authors ...show less authors
Metadata
Show full item recordAbstract
This work investigated the use of an ensemble deep random vector functional link (edRVFL) network for electroencephalogram (EEG)-based driver fatigue recognition. Against the low feature learning capability of the edRVFL network from raw EEG signals, two strategies were exploited in this work. Specifically, the first one was to exploit the advantages of the feature extractor module in CNNs, i.e., use CNN features as the input of the edRVFL network. The second one was to improve the feature learning capability of the edRVFL network. An enhanced edRFVL network named FGloWD-edRVFL was proposed, in which four enhancements were implemented, including random forest-based Feature selection, Global output layer, Weighting and entropy-based Dynamic ensemble. The proposed FGloWD-edRVFL network was evaluated on the challenging cross-subject driver fatigue recognition tasks. The results indicated that the proposed model could boost the recognition performance, significantly outperforming all strong baselines. The step-wise analysis further demonstrated the effectiveness of the proposed enhancements in the edRVFL network. 2023 The Author(s)
Collections
- Information Intelligence [98 items ]