Self-ChakmaNet: A deep learning framework for indigenous language learning using handwritten characters
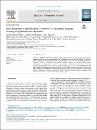
View/ Open
Publisher version (Check access options)
Check access options
Date
2023-11-22Author
Kanchon Kanti, PodderEmdad Khan, Ludmila
Chakma, Jyoti
Chowdhury, Muhammad E.H.
Dutta, Proma
Salam, Khan Md Anwarus
Khandakar, Amith
Ayari, Mohamed Arselene
Bhawmick, Bikash Kumar
Islam, S M Arafin
Kiranyaz, Serkan
...show more authors ...show less authors
Metadata
Show full item recordAbstract
According to UNESCO's Atlas of the World's Languages in Danger, 40% of the languages today are counted as endangered in the future. Indigenous languages are endangered because of the less availability of interactive learning mediums for those languages. Thus this paper proposes an interactive deep learning method for Handwritten Character Recognition of the indigenous language “Chakma.” The method comprises dataset creation using a mobile app named “EthnicData.” It reports the first “Handwriting Character Dataset” of Chakma containing 47,000 images of 47 characters of Chakma language using the app. A novel SelfONN-based deep learning model, Self-ChakmaNet, is proposed in this research for Chakma Handwritten character recognition. The Self-ChakmaNet achieved 99.84% for overall accuracy, precision, recall, F1 score, and sensitivity. The proposed model with high accuracy can be implemented in mobile devices for handwritten character recognition as the model has less number of parameters and a faster processing speed.
Collections
- Civil & Architectural Engineering [718 items ]
- Electrical Engineering [2555 items ]