Towards robust autonomous driving systems through adversarial test set generation
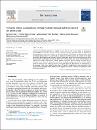
Date
2023-01-01Author
Unal, DevrimCatak, Ferhat Ozgur
Houkan, Mohammad Talal
Mudassir, Mohammed
Hammoudeh, Mohammad
Metadata
Show full item recordAbstract
Correct environmental perception of objects on the road is vital for the safety of autonomous driving. Making appropriate decisions by the autonomous driving algorithm could be hindered by data perturbations and more recently, by adversarial attacks. We propose an adversarial test input generation approach based on uncertainty to make the machine learning (ML) model more robust against data perturbations and adversarial attacks. Adversarial attacks and uncertain inputs can affect the ML model's performance, which can have severe consequences such as the misclassification of objects on the road by autonomous vehicles, leading to incorrect decision-making. We show that we can obtain more robust ML models for autonomous driving by making a dataset that includes highly-uncertain adversarial test inputs during the re-training phase. We demonstrate an improvement in the accuracy of the robust model by more than 12%, with a notable drop in the uncertainty of the decisions returned by the model. We believe our approach will assist in further developing risk-aware autonomous systems.
Collections
- Interdisciplinary & Smart Design [14 items ]