Machine learning-based prognostic model for 30-day mortality prediction in Sepsis-3
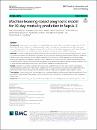
View/ Open
Date
2024Author
Rahman, Md. SohanurIslam, Khandaker Reajul
Prithula, Johayra
Kumar, Jaya
Mahmud, Mufti
Alam, Mohammed Fasihul
Reaz, Mamun Bin Ibne
Alqahtani, Abdulrahman
Chowdhury, Muhammad E. H.
...show more authors ...show less authors
Metadata
Show full item recordAbstract
Background: Sepsis poses a critical threat to hospitalized patients, particularly those in the Intensive Care Unit (ICU). Rapid identification of Sepsis is crucial for improving survival rates. Machine learning techniques offer advantages over traditional methods for predicting outcomes. This study aimed to develop a prognostic model using a Stacking-based Meta-Classifier to predict 30-day mortality risks in Sepsis-3 patients from the MIMIC-III database. Methods: A cohort of 4,240 Sepsis-3 patients was analyzed, with 783 experiencing 30-day mortality and 3,457 surviving. Fifteen biomarkers were selected using feature ranking methods, including Extreme Gradient Boosting (XGBoost), Random Forest, and Extra Tree, and the Logistic Regression (LR) model was used to assess their individual predictability with a fivefold cross-validation approach for the validation of the prediction. The dataset was balanced using the SMOTE-TOMEK LINK technique, and a stacking-based meta-classifier was used for 30-day mortality prediction. The SHapley Additive explanations analysis was performed to explain the model's prediction. Results: Using the LR classifier, the model achieved an area under the curve or AUC score of 0.99. A nomogram provided clinical insights into the biomarkers' significance. The stacked meta-learner, LR classifier exhibited the best performance with 95.52% accuracy, 95.79% precision, 95.52% recall, 93.65% specificity, and a 95.60% F1-score. Conclusions: In conjunction with the nomogram, the proposed stacking classifier model effectively predicted 30-day mortality in Sepsis patients. This approach holds promise for early intervention and improved outcomes in treating Sepsis cases.
Collections
- Electrical Engineering [2821 items ]
- Public Health [484 items ]