Evaluating the performance of metaheuristic-tuned weight agnostic neural networks for crop yield prediction
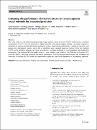
عرض / فتح
التاريخ
2024المؤلف
Jovanovic, LukaZivkovic, Miodrag
Bacanin, Nebojsa
Dobrojevic, Milos
Simic, Vladimir
Sadasivuni, Kishor Kumar
Tirkolaee, Erfan Babaee
...show more authors ...show less authors
البيانات الوصفية
عرض كامل للتسجيلةالملخص
This study explores crop yield forecasting through weight agnostic neural networks (WANN) optimized by a modified metaheuristic. WANNs offer the potential for lighter networks with shared weights, utilizing a two-layer cooperative framework to optimize network architecture and shared weights. The proposed metaheuristic is tested on real-world crop datasets and benchmarked against state-of-the-art algorithms using standard regression metrics. While not claiming WANN as the definitive solution, the model demonstrates significant potential in crop forecasting with lightweight architectures. The optimized WANN models achieve a mean absolute error (MAE) of 0.017698 and an R-squared (R2) score of 0.886555, indicating promising forecasting performance. Statistical analysis and Simulator for Autonomy and Generality Evaluation (SAGE) validate the improvement significance and feature importance of the proposed approach.
المجموعات
- الأبحاث [1449 items ]