A novel classical machine learning framework for early sepsis prediction using electronic health record data from ICU patients
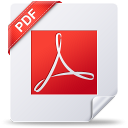
View/ Open
Publisher version (Check access options)
Check access options
Date
2025-01-31Author
Prithula, JohayraIslam, Khandaker Reajul
Kumar, Jaya
Tan, Toh Leong
Reaz, Mamun Bin Ibne
Rahman, Tawsifur
Zughaier, Susu M.
Khan, Muhammad Salman
Murugappan, M.
Chowdhury, Muhammad E.H.
...show more authors ...show less authors
Metadata
Show full item recordAbstract
Sepsis, a life-threatening condition triggered by the body's response to infection, remains a significant global health challenge, annually affecting millions in the United States alone with substantial mortality and healthcare costs. Early prediction of sepsis is critical for timely intervention and improved patient outcomes. This study introduces an innovative predictive model leveraging machine learning techniques and a specific data-splitting approach on highly imbalanced electronic health records (EHRs). Using PhysioNet/CinC Challenge 2019 data from 40,336 patients, including vital signs, lab values, and demographics. Preliminary assessments using classical and stacked ML models with Synthetic Minority Oversampling Technique (SMOTE) augmentation were conducted, showing improved performance. It is found that stacking ML models enhances overall accuracy but faces limitations in precision, recall, and F1 score for positive class prediction. A novel data-splitting approach with 5-fold cross-validation and SMOTE and COPULA augmentation techniques demonstrated promise, with F1 scores ranging from 93 % to 94 % using the COPULA technique. COPULA excelled at predictions for different hours' onsets compared to the SMOTE technique. The proposed model outperformed existing studies, suggesting clinical viability for early sepsis prediction.
Collections
- Electrical Engineering [2820 items ]
- Medicine Research [1685 items ]