Prediction of stroke-associated hospital-acquired pneumonia: Machine learning approach
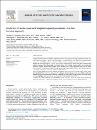
View/ Open
Publisher version (Check access options)
Check access options
Date
2025-02-28Metadata
Show full item recordAbstract
BackgroundStroke-associated Hospital Acquired Pneumonia (HAP) significantly impacts patient outcomes. This study explores the utility of machine learning models in predicting HAP in stroke patients, leveraging national registry data and SHapley Additive exPlanations (SHAP) analysis to identify key predictive factors. MethodsWe collected data from a national stroke registry covering January 2014 to July 2022, including 9,840 patients diagnosed with ischemic and hemorrhagic strokes. Five machine learning models were trained and evaluated: XGBoost, Random Forest, Support Vector Machine (SVM), Logistic Regression, and Artificial Neural Network (ANN). Performance was assessed using accuracy, precision, recall, F1-score, AUC, log loss, and Brier score. SHAP analysis was conducted to interpret model outputs. ResultsThe ANN model demonstrated superior performance, with an F1-score of 0.86 and an AUC of 0.94. SHAP analysis identified key predictors: stroke severity, admission location, Glasgow Coma score (GCS), systolic and diastolic blood pressure at admission, ethnicity, stroke type, mode of arrival, and age. Patients with higher stroke severity, dysphagia, and those arriving by ambulance were at increased risk for HAP. ConclusionThis study enhances our understanding of early predictive factors for HAP in stroke patients and underlines the potential of machine learning to improve clinical decision-making and personalized care.
DOI/handle
http://dx.doi.org/10.1016/j.jstrokecerebrovasdis.2024.108200http://hdl.handle.net/10576/64520
Collections
- Public Health [484 items ]