A new method for synthesizing test accuracy data outperformed the bivariate method
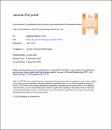
View/ Open
Publisher version (Check access options)
Check access options
Date
2020-12-01Metadata
Show full item recordAbstract
This paper outlines the development of a new method (split component synthesis; SCS) for meta-analysis of diagnostic accuracy studies and assesses its performance against the commonly used bivariate random effects model. The SCS method summarises the study-specific natural logarithm of the diagnostic odds ratios (ln(DOR)), which mainly reflects test discrimination rather than threshold effects, and then splits the summary ln(DOR) into its component parts, logit of sensitivity and logit of specificity. Performance of the estimator under the SCS method was assessed through simulation and compared against the bivariate random effects model estimator in terms of bias, mean squared error (MSE), and coverage probability across varying degrees of between-studies heterogeneity. The SCS estimator for the DOR, Se, and Sp were less biased and had smaller MSE than the bivariate model estimators. Despite the wider width of the 95% confidence intervals under the bivariate model, the latter had a poorer coverage probability compared to that under the SCS method. The SCS estimator outperforms the bivariate model estimator and thus represents an improvement in our approach to diagnostic meta-analyses. The SCS method is available to researchers through the diagma module in Stata and the SCSmeta function in R.
Collections
- Medicine Research [1518 items ]