State of charge estimation for a group of lithium-ion batteries using long short-term memory neural network
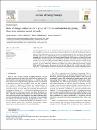
View/ Open
Publisher version (Check access options)
Check access options
Date
2022Metadata
Show full item recordAbstract
The present paper estimates for the first time the State of Charge (SoC) of a high capacity grid-scale lithium-ion battery storage system used to improve the power profile in a distribution network. The proposed long short-term memory (LSTM) neural network model can overcome the problems associated with the nonlinear battery model and adapt to the complexity and uncertainty of the estimation process. The accuracy of the developed model was compared with results obtained from Feed-Forward Neural Network (FFNN) topology and Deep-Feed-Forward Neural Network (DFFNN) topology under three different time series. The system was trained using real data from the Al-Manara PV power plant. The LSTM with learn-and-adapt-to-train-date properties, as well as the idea of "forget gate," shows exceptional ability to determine the SoC under various ID data. The LSTM properly calculated the SoC for all three-time models with a maximum standard error (MSE) of less than 0.62%, while the FFNN and DFFNN provided a fair estimate for the SoC with MSEs of 5.37 to 9.22% and 4.03 to 7.37%, respectively. The promising results can lead to excellent monitoring and control of battery management systems.
Collections
- Chemical Engineering [1182 items ]