Machine learning approaches for real-time forecasting of solar still distillate output
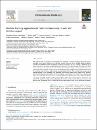
View/ Open
Publisher version (Check access options)
Check access options
Date
2023-12-01Author
Murugan, Deepak KumarSaid, Zafar
Panchal, Hitesh
Gupta, Naveen Kumar
Subramani, Sekar
Kumar, Abhinav
Sadasivuni, Kishor Kumar
...show more authors ...show less authors
Metadata
Show full item recordAbstract
Solar stills provide a promising avenue for freshwater production in regions grappling with water scarcity, especially remote locales. However, their efficiency is often constrained by the variable climatic conditions. Conventional prediction methods fall short in consistently forecasting the yield, leaving a significant gap in optimizing solar still operations. Recognizing this, the introduction of machine learning becomes pivotal. With a robust predictive model, operators can avoid inefficiencies, inconsistent outputs, and sub-optimal resource utilization. The primary objective of this research is to determine the most suitable machine learning model tailored for predicting solar still output under specific environmental conditions. This research work assessed various machine learning models, including linear regression, decision trees, random forest, support vector machines, and multilayer perceptron. Evaluation metrics encompassed Mean Absolute Error (MAE), cross-validation, grid search, and randomized search techniques. Our results identified the Decision Tree model, registering a MAE of 5.43 and 5.74 through random and grid search methods, respectively, as the preeminent predictor for our dataset. This machine learning-centric methodology elevates the precision of solar still output predictions and paves the way for enhanced solar still designs and superior optimization of solar energy conversion mechanisms.
Collections
- Center for Advanced Materials Research [1485 items ]