On reliability enhancement of solar PV arrays using hybrid SVR for soiling forecasting based on WT and EMD decomposition methods
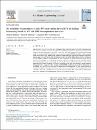
View/ Open
Publisher version (Check access options)
Check access options
Date
2024-06-01Metadata
Show full item recordAbstract
Solar farms have PV arrays in arid and semi-arid regions where ensuring the system's reliability is paramount and face uncertain events like dust storms. The deposition of random dust patterns over panel arrays is called uneven soiling, which diminishes the power generation of such farms. This paper finds the most suitable hybrid algorithm model, the wavelet transform-based support vector regression variants (WT-SVR) algorithm, and the empirical model decomposition-based support vector regression variants (EMD-SVR) to predict the extent of soiling levels and uncertain events on PV arrays. The soiling dataset is taken from NREL's Soiling Station Number 3 in Imperial County, Calipatria, California, from December 30, 2014, to December 31, 2015. This research tested four SVR variants on soiling data, viz., εSVR, LSSVR, TSVR, and εTSVR, then compared with the benchmark random forest. The hyperparameters for each model are meticulously tuned to enhance the robustness of the trained algorithms. Results reveal that the WT-TSVR model outperforms the WT-SVR model in terms of wavelet transform decomposition by a margin of 91.6%. Similarly, the EMD-TSVR model showcases an 85.7% enhancement in performance over the EMD-SVR model based on empirical mode decomposition. All SVR variants outperform the benchmark model (RF). Furthermore, EMD models exhibit enhanced efficiency in forecasting random events compared to WT, which is attributed to their reduced computational time. This model applies to multi-cleaning agent robots, aligning with recommendations from the state-of-the-art literature.
Collections
- Electrical Engineering [2840 items ]