Machine Learning Approach to Predict Metro Ridership based on Land Use Densities
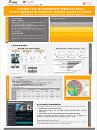
عرض / فتح
التاريخ
2021المؤلف
Alkhereibi, Aya HasanWakjira, Tadesse
kucukvar, Murat
Qidwai, Uvais
Muley, Deepti
Siam, Abdalla
...show more authors ...show less authors
البيانات الوصفية
عرض كامل للتسجيلةالملخص
Predicting metro ridership is an essential requirement for efficient metro operation and management. The dependence of metro ridership on the land use densities entails a need for an accurate predictive model. To this end, the current study is aimed to develop a novel machine learning (ML) based model to predict the metro station ridership utilizing the land use densities near metro stations. The ridership data was obtained from Qatar Rail, and the land use data were obtained from the Ministry of Municipality and Environment in Qatar. The land use densities in the catchment area of 800 m around the metro stations have been considered in this study. The non-linear relationship between the metro ridership and land use densities has been captured through different ensemble ML models including random forests, extremely randomized trees, and gradient tree boosting. Results showed that the ML models, once meticulously optimized and trained are capable of producing an accurate prediction for metro ridership. Among the ML models, gradient tree boosting showed the highest prediction capability. The authors concluded that the proposed prediction model can be utilized by both urban and transport planners in their processes to plan the land use around metro stations, predict the transit demand from those plans, and ultimately achieve the optimal use of the transit system i.e., Transit-Oriented Developments.
معرّف المصادر الموحد
https://dx.doi.org/10.29117/quarfe.2021.0164DOI/handle
http://hdl.handle.net/10576/24528المجموعات
- Theme 3: Information and Communication Technologies [16 items ]
- النقل [90 items ]