Digital twin in energy industry: Proposed robust digital twin for power plant and other complex capital-intensive large engineering systems
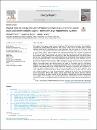
View/ Open
Publisher version (Check access options)
Check access options
Date
2022Metadata
Show full item recordAbstract
The complex future power plants require digital twin (DT) architecture to achieve high reliability, availability and maintainability at lower cost. The available research on DT for power plants is limited and lacks details on DT comprehensiveness and robustness. The main focus of the present study is to propose a comprehensive and robust DT architecture for power plants that can also be used for other similar complex capital-intensive large engineering systems. First, overviews are conducted for DT key research and development for power plants and related energy savings applications to provide current status, guidelines and research gaps. Then, the requirements and rules for the power plant DT are established and the major DT components are determined. These components include the physics-based formulations; the statistical analysis of data from the sensor network; the real-time data; the pre-performed localized in-depth simulations to predict activities of the corresponding physical twin; and the system Genome with a digital thread that connects all these components together. Recommendations and future directions are made for the power plant DT development including the need for real data and physical description of the overall system focusing on each component individually and on the overall connections. Data-driven algorithms with capabilities to predict the system's dynamic behavior still need to be developed. The data-driven approach alone is not sufficient and a low-order physics based model should operate in tandem with the updated latest system parameters to allow interpretation and enhancing the results from the data-driven process. Discrepancies between the dynamic system models (DSM) and anomaly detection and deep learning (ADL) require in-depth localized off-line simulations. Furthermore, this paper demonstrates the advantages of the developed ADL algorithm approach and DSM prediction of the DT using vector autoregressive model for anomaly detection in utility gas turbines with data from an operational power plant.
Collections
- Mechanical & Industrial Engineering [1306 items ]