Secure and efficient prediction of electric vehicle charging demand using 𝛼 2 -LSTM and AES-128 cryptography
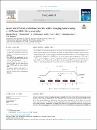
View/ Open
Publisher version (Check access options)
Check access options
Date
2024-05-01Author
Bharat, ManishDash, Ritesh
Reddy, K. Jyotheeswara
Murty, A. S.R.
C., Dhanamjayulu
Muyeen, S. M.
...show more authors ...show less authors
Metadata
Show full item recordAbstract
In recent years, there has been a significant surge in demand for electric vehicles (EVs), necessitating accurate prediction of EV charging requirements. This prediction plays a crucial role in evaluating its impact on the power grid, encompassing power management and peak demand management. In this paper, a novel deep neural network based on α2 -LSTM is proposed to predict the demand for charging from electric vehicles at a 15-minute time resolution. Additionally, we employ AES-128 for station quantization and secure communication with users. Our proposed algorithm achieves a 9.2% reduction in both the Root Mean Square Error (RMSE) and the mean absolute error compared to LSTM, along with a 13.01% increase in demand accuracy. We present a 12-month prediction of EV charging demand at charging stations, accompanied by an effective comparative analysis of Mean Absolute Percentage Error (MAPE) and Mean Percentage Error (MPE) over the last five years using our proposed model. The prediction analysis has been conducted using Python programming.
Collections
- Electrical Engineering [2709 items ]