Budgeted online selection of candidate iot clients to participate in federated learning
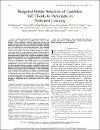
Date
2021-04-01Author
Mohammed, IhabTabatabai, Shadha
Al-Fuqaha, Ala
Bouanani, Faissal El
Qadir, Junaid
Qolomany, Basheer
Guizani, Mohsen
...show more authors ...show less authors
Metadata
Show full item recordAbstract
Machine learning (ML), and deep learning (DL) in particular, play a vital role in providing smart services to the industry. These techniques, however, suffer from privacy and security concerns since data are collected from clients and then stored and processed at a central location. Federated learning (FL), an architecture in which model parameters are exchanged instead of client data, has been proposed as a solution to these concerns. Nevertheless, FL trains a global model by communicating with clients over communication rounds, which introduces more traffic on the network and increases the convergence time to the target accuracy. In this work, we solve the problem of optimizing accuracy in stateful FL with a budgeted number of candidate clients by selecting the best candidate clients in terms of test accuracy to participate in the training process. Next, we propose an online stateful FL heuristic to find the best candidate clients. Additionally, we propose an IoT client alarm application that utilizes the proposed heuristic in training a stateful FL global model based on IoT device-type classification to alert clients about unauthorized IoT devices in their environment. To test the efficiency of the proposed online heuristic, we conduct several experiments using a real data set and compare the results against state-of-the-art algorithms. Our results indicate that the proposed heuristic outperforms the online random algorithm with up to 27% gain in accuracy. Additionally, the performance of the proposed online heuristic is comparable to the performance of the best offline algorithm.
Collections
- Computer Science & Engineering [2428 items ]