Thermal Change Index-Based Diabetic Foot Thermogram Image Classification Using Machine Learning Techniques
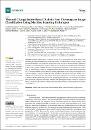
View/ Open
Date
2022Author
Khandakar, AmithChowdhury, Muhammad E. H.
Reaz, Mamun B.
Ali, Sawal H.
Abbas, Tariq O.
Alam, Tanvir
Ayari, Mohamed A.
Mahbub, Zaid B.
Habib, Rumana
Rahman, Tawsifur
Tahir, Anas M.
Bakar, Ahmad Ashrif A.
Malik, Rayaz A.
...show more authors ...show less authors
Metadata
Show full item recordAbstract
Diabetes mellitus (DM) can lead to plantar ulcers, amputation and death. Plantar foot thermogram images acquired using an infrared camera have been shown to detect changes in temperature distribution associated with a higher risk of foot ulceration. Machine learning approaches applied to such infrared images may have utility in the early diagnosis of diabetic foot complications. In this work, a publicly available dataset was categorized into different classes, which were corrobo-rated by domain experts, based on a temperature distribution parameter-the thermal change index (TCI). We then explored different machine-learning approaches for classifying thermograms of the TCI-labeled dataset. Classical machine learning algorithms with feature engineering and the convolutional neural network (CNN) with image enhancement techniques were extensively investigated to identify the best performing network for classifying thermograms. The multilayer perceptron (MLP) classifier along with the features extracted from thermogram images showed an accuracy of 90.1% in multi-class classification, which outperformed the literature-reported performance metrics on this dataset. 2022 by the authors. Licensee MDPI, Basel, Switzerland.
Collections
- Civil and Environmental Engineering [860 items ]
- Electrical Engineering [2821 items ]