IDRISI-RE: A generalizable dataset with benchmarks for location mention recognition on disaster tweets
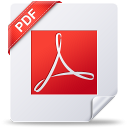
View/ Open
Publisher version (Check access options)
Check access options
Date
2023Metadata
Show full item recordAbstract
While utilizing Twitter data for crisis management is of interest to different response authorities, a critical challenge that hinders the utilization of such data is the scarcity of automated tools that extract geolocation information. The limited focus on Location Mention Recognition (LMR) in tweets, specifically, is attributed to the lack of a standard dataset that enables research in LMR. To bridge this gap, we present IDRISI-RE, a large-scale human-labeled LMR dataset comprising around 20.5k tweets. The annotated location mentions within the tweets are also assigned location types (e.g., country, city, street, etc.). IDRISI-RE contains tweets from 19 disaster events of diverse types (e.g., flood and earthquake) covering a wide geographical area of 22 English-speaking countries. Additionally, IDRISI-RE contains about 56.6k automatically-labeled tweets that we offer as a silver dataset. To highlight the superiority of IDRISI-RE over past efforts, we present rigorous analyses on reliability, consistency, coverage, diversity, and generalizability. Furthermore, we benchmark IDRISI-RE using a representative set of LMR models to provide the community with baselines for future work. Our extensive empirical analysis shows the promising generalizability of IDRISI-RE compared to existing datasets. We show that models trained on IDRISI-RE better tackle domain shifts and are less susceptible to change in geographical areas.
Collections
- Computer Science & Engineering [2129 items ]