Rapid wall shear stress prediction for aortic aneurysms using deep learning: a fast alternative to CFD
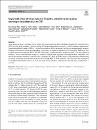
View/ Open
Date
2025-02-17Author
Faisal, Md Ahasan AtickMutlu, Onur
Mahmud, Sakib
Tahir, Anas
Chowdhury, Muhammad E.H.
Bensaali, Faycal
Alnabti, Abdulrahman
Yavuz, Mehmet Metin
El-Menyar, Ayman
Al-Thani, Hassan
Yalcin, Huseyin Cagatay
...show more authors ...show less authors
Metadata
Show full item recordAbstract
Abstract: Aortic aneurysms pose a significant risk of rupture. Previous research has shown that areas exposed to low wall shear stress (WSS) are more prone to rupture. Therefore, precise WSS determination on the aneurysm is crucial for rupture risk assessment. Computational fluid dynamics (CFD) is a powerful approach for WSS calculations, but they are computationally intensive, hindering time-sensitive clinical decision-making. In this study, we propose a deep learning (DL) surrogate, MultiViewUNet, to rapidly predict time-averaged WSS (TAWSS) distributions on abdominal aortic aneurysms (AAA). Our novel approach employs a domain transformation technique to translate complex aortic geometries into representations compatible with state-of-the-art neural networks. MultiViewUNet was trained on 23 real and 230 synthetic AAA geometries, demonstrating an average normalized mean absolute error (NMAE) of just 0.362% in WSS prediction. This framework has the potential to streamline hemodynamic analysis in AAA and other clinical scenarios where fast and accurate stress quantification is essential.
Collections
- Biomedical Research Center Research [776 items ]
- Biomedical Sciences [784 items ]
- Electrical Engineering [2821 items ]
- Mechanical & Industrial Engineering [1462 items ]