Novel and robust machine learning approach for estimating the fouling factor in heat exchangers
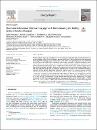
View/ Open
Publisher version (Check access options)
Check access options
Date
2022Author
Hosseini, SalehKhandakar, Amith
Chowdhury, Muhammad E.H.
Ayari, Mohamed Arselene
Rahman, Tawsifur
Chowdhury, Moajjem Hossain
Vaferi, Behzad
...show more authors ...show less authors
Metadata
Show full item recordAbstract
The fouling factor (Rf) is an operating index for measuring an undesirable effect of solids’ deposition on
the heat transfer ability of heat exchangers. Accurate prediction of the fouling factor helps appropriate
scheduling of the cleaning cycles. Since diverse factors affect this operating feature, it is sometimes
hard to estimate the fouling factor accurately using simple empirical or traditional intelligent meth-
ods. Therefore, this study employs four up-to-date machine-learning algorithms (Gaussian Process
Regression, Decision Trees, Bagged Trees, Support Vector Regression) and a traditional model (Linear
Regression) to estimate the fouling factor as a function of operating and constructing variables. The 5-
fold cross-validation using 9268 data samples determines the structure of the considered estimators,
and 2358 external datasets have been utilized for models’ testing. The relevancy analysis confirms
that the most accurate predictions are achieved when the square root of the fouling factor (√Rf)
is simulated. The Gaussian Process Regression (GPR) shows the highest level of agreement with the
experimental samples in both the model construction and testing stages. The trained GPR model scored
an R2 value of 0.98770 and 0.99857 on the internal and external datasets, respectively. The model
predicts the overall 11626 experimental samples (Davoudi and Vaferi, 2018) with the MAPE = 13.89%,
MSE = 7.02 × 10−4, and R2 = 0.98999. The proposed GPR model outperforms the previously suggested
artificial neural network for estimating the fouling factor in heat exchangers.
Collections
- Civil and Environmental Engineering [851 items ]
- Electrical Engineering [2649 items ]
- Technology Innovation and Engineering Education Unit [63 items ]